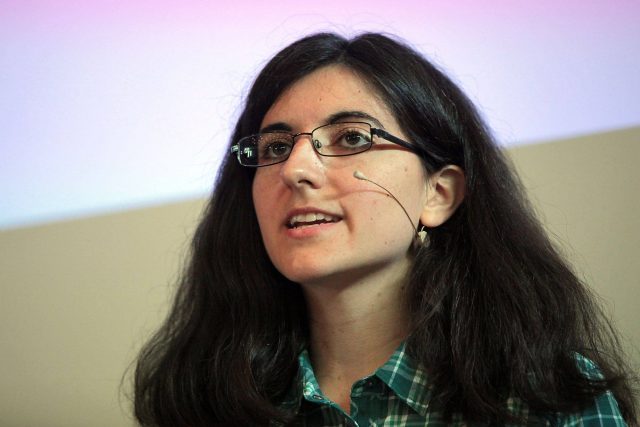
Tamara Broderick featured by “Women in Machine Learning”
Tamara Broderick is the ITT Career Development Assistant Professor at MIT. At MIT, she is a member of the Electrical Engineering and Computer Science Department, the Computer Science and Artificial Intelligence Laboratory, and the Institute for Data, Systems, and Society. She creates streaming, distributed methods to unite Bayesian models and big data. She writes:
“The promise of Big Data isn’t simply to estimate a mean with greater accuracy; practitioners are interested in learning complex information and interactions from large data sets. Bayesian statistics allows not only flexible modeling but also a coherent treatment of model uncertainty as data accrue. Moreover, nonparametric Bayes goes a step further by providing models whose complexity grows with the size of the data. We expect to see, e.g., a greater diversity of topics as we read more documents from the New York Times or more social groups as we process more of Facebook’s network structure.
“The output of a Bayesian analysis (parametric or nonparametric) is the Bayesian posterior distribution. For complex problems, the posterior cannot be calculated exactly, and much work has focused on delivering an accurate posterior approximation. But the computational cost of these approximations can sometimes be prohibitive.
“In a series of projects, I’ve been working on the idea that sometimes we’re willing to trade off some knowledge of the posterior for computational gains. At one extreme, I developed a method ‘MAD-Bayes’ that turns complex nonparametric Bayesian models into simple-to-use, K-means-like objective functions. It lets us get fast point estimates, but at the cost of accurate uncertainty estimates. MAD-Bayes has been used in a number of biological applications, including the analysis of tumors that may exhibit multiple types of cancer.
“On the other end, I’ve worked on improving mean-field variational Bayes (MFVB), a popular and fast posterior approximation method that is known to provide poor estimates of both individual parameter uncertainty and covariance between parameters. Uncertainty is hugely important in many applications; you might make a very different investment if you knew the return would be exactly $20 vs if you knew the return would be $20 with a standard deviation of $10K. My collaborators and I have extended the basic MFVB algorithm to a version that can make use of modern, distributed architectures and work on streaming data—and also developed an augmentation to MFVB that delivers accurate estimates of posterior uncertainty for model parameters.”