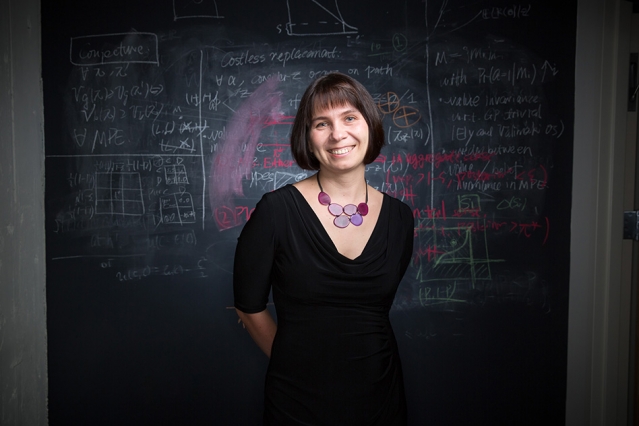
Timing devices
Ask enough scholars to explain their work, and sometimes surprising descriptions will emerge.
“My thesis was on the estimation of persistence, how much you remember from the past,” MIT Associate Professor Anna Mikusheva says of her PhD dissertation.
It may sound like Mikusheva is a psychologist or a Proust scholar. But she’s an econometrician: a scholar who studies the techniques of estimation used in economic models. More precisely, Mikusheva specializes in time-series econometrics, the subdiscipline that examines sequences of observations pertaining to a particular statistical output over time: inflation, gross domestic product, stock prices, and so on.
So when Mikusheva talks about “how much you remember,” she is being metaphorical about the key issue in time-series econometrics: To what extent are these discrete data points, like the history of a stock price, connected across time?
“Time series is exactly about time dependence, how different observations depend on each other,” Mikusheva explains.
Her work both analyzes existing economics tools in the domain, and helps to develop new techniques that can be applied to time-series problems. To fully account for the trajectory of something like inflation, of course, you need a thorough explanation of the various factors involved. But econometrics can help us see how solid such models are statistically — and how likely there is to be persistence and continuity in the processes that churn out economic data. For her innovative work and her teaching, Mikusheva was granted tenure at MIT earlier this year.
Passing the test
As it happens, Mikusheva’s personal journey, from a childhood in the Soviet Union to her position at MIT, is a time series with plenty of discontinuities in it. Among other things, she didn’t study economics as a student growing up in Orenburg, a Russian city in the Ural Mountains, near the border of Kazakhstan.
“It just happened that I liked math, from the beginning, from childhood,” Mikusheva says. “My teachers pushed me.” As she recalls it, her family provided additional moral support, though not necessarily academic direction.
“My parents were very supportive people who believed in education, but they didn’t know much about how education works, so they didn’t have much experience to know what to do,” Mikusheva says. “I had a very good teacher in math who thought that I had talent, so she pushed me to the Math Olympics, and so I competed a little bit on the regional level.”
Participating in the Math Olympics at age 15, Mikusheva didn’t get any farther than the regional level, but she caught a break while competing: Scouts from a boarding school in Moscow noticed her and offered her a spot at their academy, if she could pass the entrance exam.
Mikusheva passed the test, left home, and attended the school. “It was a rare opportunity,” she says.
Mikusheva then continued to Moscow State University, where she received her undergraduate degree in mathematics in 1998. Mikusheva began focusing formally on economics while receiving an MA at Moscow’s New Economic School and simultaneously finishing a PhD in math at Moscow State.
As it turned out, her graduate education was only about half-finished: She did well enough as a graduate student to enter Harvard University’s PhD program in economics. She entered intending to study game theory, but moved over to time-series econometrics, partly because her advisor, James Stock, had identified some problems Mikusheva thought she could address.
“It felt more natural to do econometrics,” Mikusheva reflects. “It’s mathematical statistics. I said, ‘That’s probably where my strength is.’”
When “the data does not speak as loudly”
Mikusheva was right: She finished her PhD in 2007, and her thesis, on a concept she developed known as “uniform asymptotic approximation,” was quickly published in a top journal. Many statistical techniques used for the evaluation of economic models are based on justifications assuming that the amount of data involved can grow infinitely. But economic data are not infinite, so how accurately do classical statistical methods perform when applied to real-world data? Mikusheva’s method shows where specific parts of models’ estimations might be less reliable than others — and indicates that a couple of common statistical methods in economics run into this reliability problem.
“As econometricians we are not developing models per se, but we are trying to develop methods that could be used by applied researchers, and I think as econometricians we do care about the quality of the methods we provide,” she says.
Since her initial success, Mikusheva has pursued multiple lines of research, with some converging around the notion of “weak identification” — the problem of having on a scarcity of information relating to part of an economic model. This is a particular problem for some macroeconomic (or large-scale) models, including those known as dynamic stochastic general equilibrium (DSGE) models.
“I have high opinions about my colleagues in other fields [of economics],” Mikusheva says. “Solving big macro models is quite a challenge, and do they do this quite successfully.” That said, she adds, “The difficulty with DSGE models is that data do not speak as loudly as we would wish.”
Now several years into her career, Mikusheva emphasizes how pleased she is to have landed at MIT.
“The department has been a very supportive environment,” Mikusheva observes. “It’s known to be one of the most cooperative departments. People like talking with each other.”
For Mikusheva, productive conversations also occur in the classroom: “I love teaching,” she says. “Sometimes [the students] teach me more than I teach them. I love discussion in class, and they often ask very interesting questions.”
For her efforts, Mikusheva was granted the MIT Economics Graduate Teacher of the Year award, in 2008. For her research, Mikusheva received the Elaine Bennett Research Prize from the American Economics Association in 2012, given biannually to a woman in economics who is within seven years of receiving her PhD.
In the immediate future, Mikusheva has more papers on weak identification in the publication pipeline, and she is continually looking at new areas of econometrics to explore. But whether there will be strong continuities between her past and future research — well, time will tell.