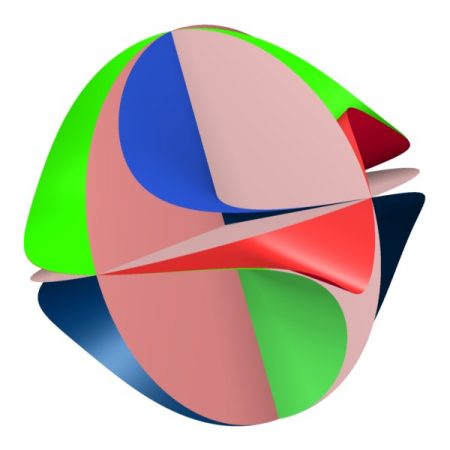
Causal inference and applications to learning gene regulatory networks
Causal inference is a cornerstone of scientific discovery because it seeks to determine causative relationships between variables. It is of particular interest to determine causal structure among variables based on observational data, since conducting randomized controlled trials is often impractical or prohibitively expensive. An exciting and quite recent application of causal inference is to infer gene regulatory networks. The interaction between genetics applications and causal statistical methodology carries a particularly beneficial symbiosis. Genes themselves are always causes and never effects. In addition, geneticists are able to perform interventional experiments relatively easily; these can be used to validate causal inferences, making this area an ideal test bed for new statistical methodology. In our work we establish limitations of various existing algorithms for causal inference, use the geometry of conditional independence structures to develop new algorithms, and we are currently applying these new algorithms to learn gene regulatory networks in space (different cell types) and time (throughout development).
Caroline Uhler
Professor, Electrical Engineering and Computer Science
Selected Publications
G. Raskutti and C. Uhler. Learning directed acyclic graphs based on sparsest permutations. Submitted.
A. Klimova, C. Uhler, and T. Rudas. Faithfulness and learning of hypergraphs from discrete distributions. Journal of Computational Statistics and Data Analysis, 87:57–72, 2015.
S. Lin, C. Uhler, B. Sturmfels, and P. Bühlmann. Hypersurfaces and their singularities in partial correlation testing. Foundations of Computational Mathematics, 14:1079-1116, 2014.
C. Uhler, G. Raskutti, P. Bühlmann, and B. Yu. Geometry of faithfulness assumption in causal inference. Annals of Statistics 41:436–463, 2013.