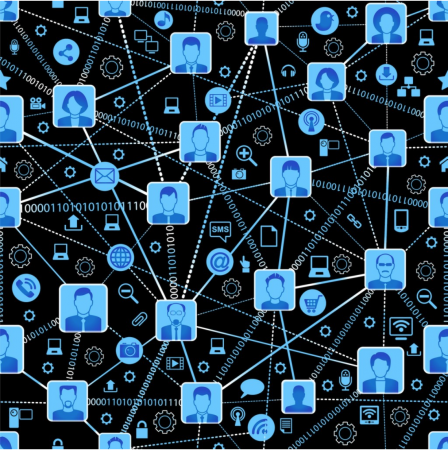
Online Learning
“Consider the following basic question: given two populations (arms), at each time t=1,…,T, sample from only one of the populations and receive a random reward dictated by the properties of the sampled population. The objective is to devise a policy that maximizes the expected cumulative reward over the T rounds. While the terminology “arm” comes from a well known analogy with slot machines, the original motivation for this question comes from clinical trials where each arm corresponds to a treatment and rewards measure the efficacy of this treatment on a patient. When devising a strategy to solve this problem, one faces the so-called exploration versus exploitation dilemma. Indeed, there is a clear tradeoff between discovering which treatment is the most effective (exploration) and administering the best treatment to as many patients as possible (exploitation). Such problems are of particular importance in online advertising where customers arrive sequentially at a fast rate. In this line of research, we develop strategies to optimize utility in this dynamic environment in an optimal fashion.”
Philippe Rigollet
MIT Assistant Professor, Department of Mathematics
Selected Publications
J. Weed, V. Perchet and P. Rigollet. Online learning in repeated auctions. Submitted.
V. Perchet, P. Rigollet, S. Chassang and E. Snowberg. Batched Bandit Problems. Annals of Statistics. 2015.
V. Perchet and P. Rigollet. Bounded regret in stochastic multi-armed bandits. Conference on Learning Theory. 2013.
V. Perchet and P. Rigollet. The multi-armed bandit problem with covariates, Annals of Statistics. 2015.