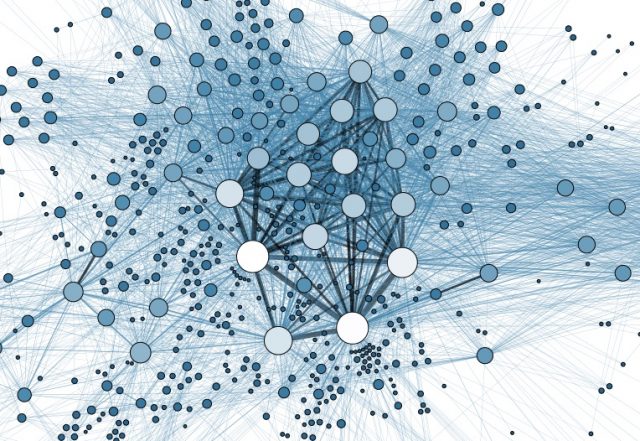
Statistical and Computational Tradeoffs
“Computational limitations of statistical problems have largely been ignored or simply overcome by ad hoc relaxations techniques. If optimal methods cannot be computed in reasonable time, what is the best possible statistical performance of a computationally efficient procedure? In our papers on sparse PCA, we have redefined the classical notion of optimality to incorporate the computational aspects of statistical methods and showed the existence of a gap between the old and the new notion. This is the first result of its kind and it opens the door for a much better understanding of many more statistical problems, especially in high dimensions. In particular, it allows a better discrimination between statistical procedures that are currently sorted out using ad-hoc and often unrealistic assumptions.
Practically, our approach relies on the use of polynomial time reductions. While such ideas have been developed by theoretical computer scientists for decades, virtually all the current literature on reductions is about worst case complexity, while statistical problems fall naturally in the framework of average case complexity. Unfortunately, the latter is still poorly understood, as current reduction techniques tend to break the fragile distributional assumptions attached to a computational problem. Building on insight from statistics, we have introduced a notion of robustness that allows us to bypass this limitation and, for the first time, to present an average case reduction between two problems that are not artificial.
Looking forward, we aim to (i) push this analysis to other high-dimensional statistical problems and (ii) develop a toolbox to derive computational lower bounds in a more systematic fashion.”
Philippe Rigollet
MIT Assistant Professor, Department of Mathematics
Selected Publications
Q. Berthet and P. Rigollet. Complexity Theoretic Lower Bounds for Sparse Principal Component Detection. Conference on Learning Theory. 2013.
Q. Berthet and P. Rigollet. Optimal detection of sparse principal components in high dimension. 2013.